Learning analytics has been around for decades, but could it be about to finally live up to the hype?
In the past six months, the swift rise of technologies such as ChatGPT, DALL-E, Midjourney, and other forms of Generative AI have underscored the profound impact of AI, extending beyond education to influence our wider world. My hunch is that most universities are grappling with a sense of lagging behind the accelerating pace of change, and this is because we are witnessing unprecedented disruption with the dawn of the 4th Industrial Revolution.
The crucial question we must address is how higher education can maintain its relevance in this forthcoming era. Higher education leaders need to consider how they can position their institution as a global contender during the 4th Industrial Revolution.
One legacy issue we face from the era of electronics and IT is the glut of information at our disposal. This overabundance gives rise to the notion that machine learning can serve as a tool to ‘penetrate the fog’ as Phil Long and George Seimens famously put it. So how can Learning Analytics help?
Key Benefits
We’ve understood for quite some time the manifold benefits of analytics. These include early problem identification and providing students with enhanced performance feedback, creating more personalized learning experiences with tailored content. Additionally, analytics play a crucial role in refining our teaching strategies and enabling us to design more effective, student-centric courses.
What’s the value proposition?
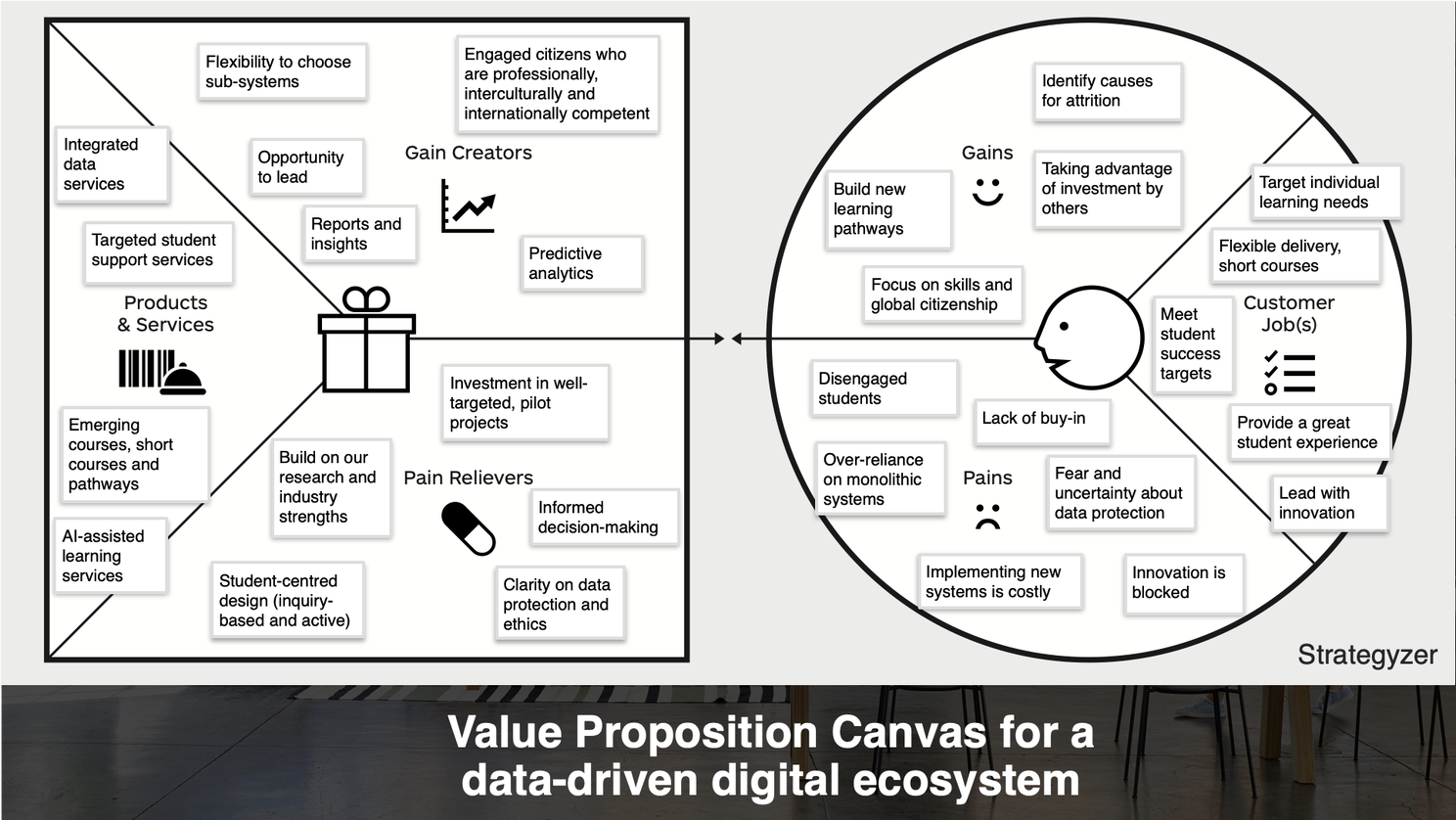
If we consider the view of a university planning for a data-driven digital ecosystem, we can consider some of the key objectives, like meeting students’ individual learning needs, improving flexibility, or creating a great student experience. The value proposition canvas in Figure 1 is just an example. You can imagine targeting products and services that use these data to provide support where it’s needed most, or even target course creation in areas of demand.
Some Risks
Leveraging student data in this way is certainly not without its risks, however. Recently, I attended the HEPI annual lecture, where Andreas Schleicher from the OECD discussed global trends in higher education. There were numerous insights, for example: by 2043, the OECD population aged 20-24 will decline by 3% compared to 2023, and in The Netherlands, this population will shrink by approximately 15%. Schleicher acknowledged the value of learning analytics in providing targeted student support but also highlighted the risk to student confidentiality.
Here are some additional key risks that need our attention:
- Legal/Privacy: GDPR compliance, including data security, consent, transparency, and data retention.
- Ethical: The potential for bias or misuse of data for unintended purposes. We must ensure that marginalized groups are accurately represented.
- Financial: The cost of projects and technology. Many learning analytics initiatives come with hefty price tags and deliver minimal return.
- People: Ensuring buy-in and overcoming risk aversion. While it may sound counter-intuitive, we need to overcome this aversion to realize our objectives.
- Technology: Keeping pace with rapid technological advancements, such as machine learning. There’s a significant risk that the current generation of ML will be surpassed by large language models in the solution space of learning analytics.
Case study 1: The Ontask Project
Simon Buckingham Shum’s work at the University of Technology, Sydney, is renowned in Australia. He was involved in a nationally-funded OLT project known as ‘The OnTask Project‘. Its primary objective was to enhance the academic experience of students through the delivery of timely, personalised, and actionable student feedback throughout their course participation.
Feedback is an essential component of successful learning. I’d argue that this “intervention” approach is a robust, generally applicable model we could aim for.
This model involves ensuring that institutional data sources feed into a central student matrix and that certain rules and actions are triggered under particular circumstances. For instance, if the system identifies that a student is struggling in a specific area, it might trigger an email to the student. Alternatively, an action could be initiated in a CRM for follow-up. This is a promising approach.
Case study 2: The University of Surrey
An excellent example of utilising LMS data is demonstrated by the University of Surrey. They employ D2L Brightspace’s core analytics package, the Brightspace Data Hub.
They linked this to individual data sets and engaged D2L’s data consultancy team to custom-build a tool that connects to the Data Hub. The data encompasses elements like student grades, content, and discussion forums, and allows Surrey to ‘nudge’ students to improve their success. Using this model, the team can export data via CSV or establish a connection to the Data Hub via API.
All this was possible without adding more advanced tools like D2L’s Performance+ tools for BrightsSpace. This provides dashboards for visualisations, an insights report builder, a predictive analytics tool, and a personalised learning pathways tool named LEAP. Institutions need to consider a cost-benefit analysis, and I would recommend considering these tools once they have a robust understanding of needs.
How might Generative AI Tools change the game?
A new generation of AI tools based on large language models creates a wealth of opportunities for learning analytics and arguably may supersede some existing AI tools that have been used for Learning Analytics until now. There is a potential power of generative tools being in the hands of learners themselves, in that this can foster a sense of agency and creativity that has perhaps eluded some of the systems that have oftentimes been more focused on features like reporting and visualisation rather than personalised learning or individual feedback. For example, students may be notified when they are dropping behind, and given some generic resources using today’s tools, whereas GenerativeAI tools can give you more direct feedback on what you can improve.
An example is the new Automated Feedback tool recently launched by Feedbackfruits, which uses generative AI to do smart things like suggest structural improvements, citation formats, and appropriate grammar for academic writing, leaving room for higher-order thinking feedback to the experts. Feedback is one of those things that learners always crave, and switching these tools on seems likely to create a lot more value than just letting them know where they sit in relation to their class.
This is just the tip of the iceberg for the future of Learning Analytics using new AI tools like OpenAI’s APIs for GPT4, with an API for Google Bard currently in beta and many other LLMs in development. If the challenges above such as confidentiality and privacy can be overcome, turning these tools on the huge data repositories generated by learning environments is likely to provide speedy and timely insights that were difficult or expensive in the past.
About the author
I am an independent consultant focusing on improving learners’ lives using emerging educational technologies and learning space design (physical and virtual). If you would like to book a 30-minute consultation, please visit my website: RocketShoes.io.